Matplotlib is a powerful Python library that helps to understand data in a visual way. This tool is very important in algo trading, crypto trading and stock market algorithms. It not only makes data analysis easier, but also makes it easier to create strategies.
Scatter plot: Show the relationship of data
If you want to understand the relationship between two variables such as price and profit, then the scatter plot is the best way. In this, each point represents a value pair, which makes it easy to see the trend.
import matplotlib.pyplot as plt
# Load the data
df = pd.read_csv(‘data.csv’)
# Create the scatter plot
plt.figure(figsize=(10, 6))
plt.scatter(df[‘Price’], df[‘Earnings’])
plt.title(‘Relationship between Price and Earnings’)
plt.xlabel(‘Price’)
plt.ylabel(‘Earnings’)
plt.show()
Bar chart: Comparison made easy
When we have to compare investments in different sectors or stocks, then the bar chart is useful. It is simple and effective. For example, you can easily compare investments in technology, healthcare and finance sectors.
# Prepare the data
sectors = [‘Technology’, ‘Healthcare’, ‘Finance’]
investment = [50000, 30000, 40000]
# Create the bar chart
plt.figure(figsize=(8, 5))
plt.bar(sectors, investment)
plt.title(‘Investment in different sectors’)
plt.xlabel(‘Sector’)
plt.ylabel(‘Investment amount’)
plt.show()
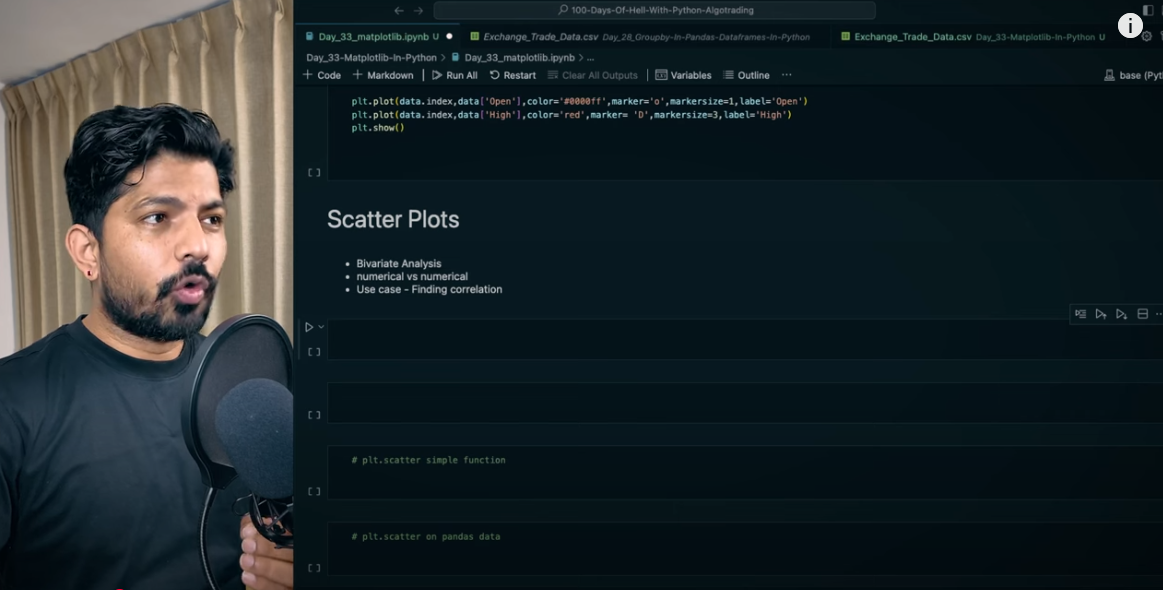
Histogram: Understand the distribution of data
If you want to see in which range the stock returns fall more, then the histogram is perfect. This will tell you which value has occurred the most.
# Stock returns data
returns = df[‘Returns’]
# Create a histogram
plt.figure(figsize=(9, 6))
plt.hist(returns, bins=20, edgecolor=’black’)
plt.title(‘Distribution of Stock Returns’)
plt.xlabel(‘Returns’)
plt.ylabel(‘Frequency’)
plt.show()
Pie Chart: Portfolio Distribution
Pie charts show how your portfolio is divided into different parts. Stocks, bonds, cash and real estate – the percentage of all is easily understood.
# Portfolio data
assets = [‘stocks’, ‘bonds’, ‘real estate’, ‘cash’]
allocation = [50, 20, 20, 10]
# Create a pie chart
plt.figure(figsize=(7, 7))
plt.pie(allocation, labels=assets, autopct=’%1.1f%%’, startangle=140)
plt.title(‘Portfolio Allocation’)
plt.show()
Customization: Make the chart special
Matplotlib gives you many ways to make the graph beautiful and informative. You can adjust the color, line style, annotation and layout as per your requirement. This makes the data understandable even better.
Colors and styles: Plots can be made distinctive by using different colors and line styles.
plt.plot(x, y, color=’green’, linestyle=’–‘, marker=’o’)
Grid and background: Adding grid lines to the graph makes it easier to read.
plt.grid(True)
Annotations: Annotations can be used to highlight particular data points.
plt.annotate(‘significant point’, xy=(x_point, y_point), xytext=(x_text, y_text),
arrowprops=dict(facecolor=’red’, shrink=0.05))
Proper data visualization is essential for algorithmic trading and crypto trading strategies. Quantitative traders can catch market trends quickly with the help of Matplotlib. This tool gives you clear direction while creating a stock algorithm. This library is also used extensively in Freqtrade tutorials.
Watch this Day 34 video tutorial
Day 34: Matplotlib In Python Part 2